A Short Note on Evaluating RepNet for Temporal Repetition Counting in Videos

By Naomi Wilson
Posted on: November 15, 2024
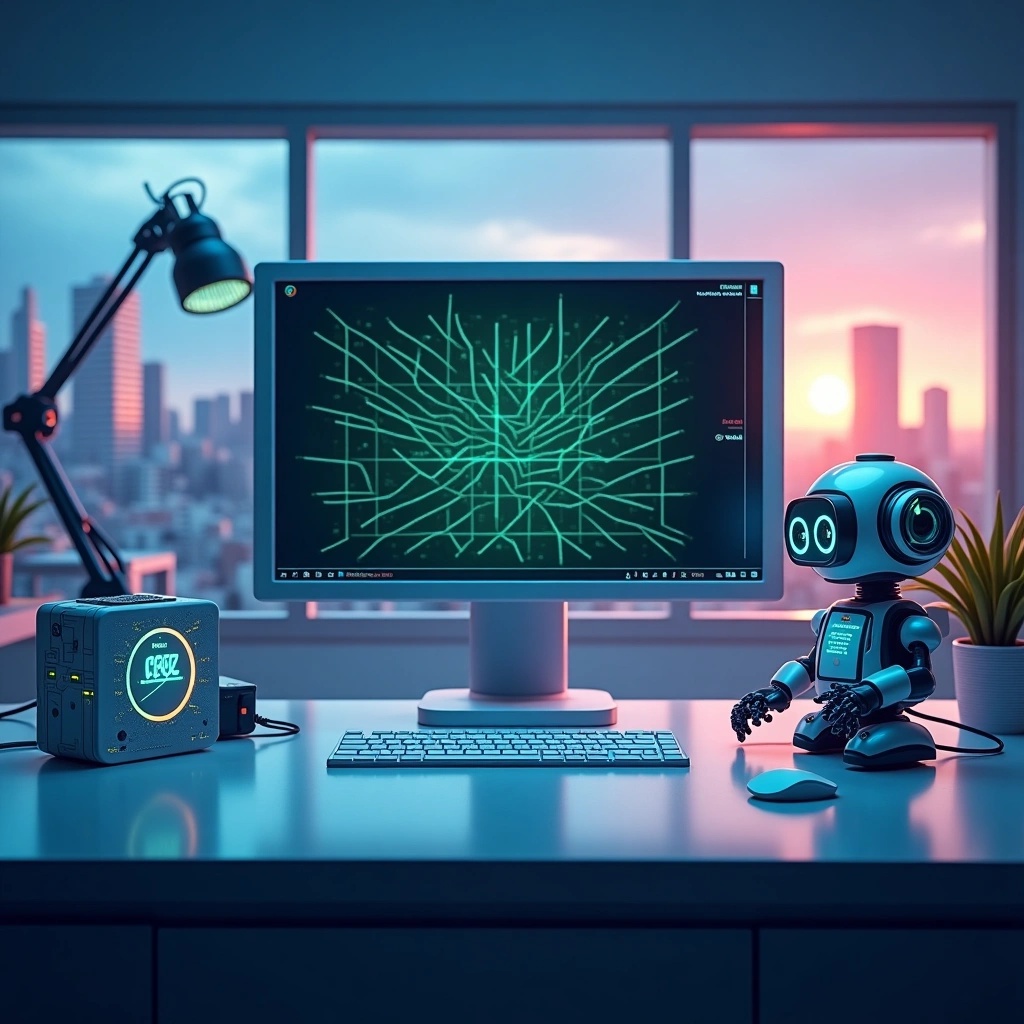
**Analyzing the Abstract**
The abstract presents a research note that aims to address inconsistencies in evaluating RepNet, a neural network architecture designed for temporal repetition counting in videos.
**What the Paper is Trying to Achieve:**
The authors identify issues with how RepNet has been evaluated in previous papers and propose a more accurate evaluation methodology. By releasing their code and checkpoint, they provide a consistent way to replicate their results and encourage further research on this topic.
**Potential Use Cases:**
1. **Video Analysis:** Temporal repetition counting is crucial in video analysis applications like action recognition, scene understanding, and anomaly detection.
2. **Surveillance Systems:** Accurate repetition counting can improve surveillance systems by detecting repetitive patterns, such as vehicles entering or leaving a parking lot.
3. **Medical Imaging:** In medical imaging, temporal repetition counting can help identify recurring patterns in medical conditions, like tumor progression.
**Significance in the Field of AI:**
1. **Consistent Evaluation:** The paper highlights the importance of consistent evaluation methods in neural network architectures to ensure reliable comparisons and advancements in the field.
2. **Improving RepNet's Performance:** By releasing their code and checkpoint, the authors provide a foundation for future research on improving RepNet's performance, which can lead to more accurate video analysis and surveillance systems.
**Papers with Code Post:**
The paper is available on Papers with Code, a platform that provides open-source code and data accompanying AI research papers. This allows researchers and practitioners to easily access and build upon the authors' work.
**Link:** https://paperswithcode.com/paper/a-short-note-on-evaluating-repnet-for-temporal
Overall, this paper's contributions will facilitate more accurate evaluations of RepNet and encourage further innovation in video analysis and surveillance systems.